By: Madeleine “Mac” Gagné
Studying Under: Dr. Hubert Bray, Duke University Mathematics
Abstract
The following research will attempt to construct a quantitative model that help sasses the social and behavioral strategies of a revolutionary situation with particular focus on alliances, the strength, and the lifetime of a regime. The research will begin by discussing the evolutionary behavioral observational studies that inspired the creation of the model. A quantitative model will then be developed. This quantitative model will include new win and points systems as well as various coefficients to track the number of follows a new regime has and how strong the regime is at any given point in time. The quantitative model will then wrap up with an outline of a revolutionary inspired game entitled The Game of Revolution. Throughout the paper, ties to evolutionary game theory and current day politics will be drawn. The study will conclude with a going forward section discussing the many current day potential applications the quantitative model possesses.
Behavioral Inspiration: Analysis of Defense Strategies in Varecia rubra
My interest in studying the evolution of defense strategies began early in the summer of 2019 when I sent in a project proposal to the Duke Lemur Center. Being interested in game theory, I wanted to know if there was an evolutionary foundation for many of the defense-based strategies in the human world today. By studying non-human primates and how the conducted themselves in defensive situations, I wanted to understand how humans had evolved to be the defensive decision makers they are today. Later on in my game theory studies, I would come to find that there exists a sub field of strategy entitled Evolutionary Game Theory whose benefit is to bring evolutionary understanding to the strategies of today’s human world. Ultimately, the study I embarked upon became one that, while unknowingly at the time, fell under the field of Evolutionary Game Theory. The proposal discussed a project in which I would observe interspecies conflicts in between groups of lemurs as a way to better understand the development of defense strategies developed in non-human primates. My intention was to utilize my findings to create a quantitative game that would exhibit the strategies I had observed in non-human primates. My proposal was approved, and I began my study in June of 2019.
Throughout June and half of July, I would visit the center three to four times a week in between the hours of 8:00 AM and 4:30 PM. I conducted two sets of 2.5-hourobservations daily. Upon arriving at the lemur center, Dr. Erin Ehmke directed me toward the center’s eight most conflict-prone groups. In total, I spent 4observational sessions of 2.5-hours with each of these groups, learning about the many defense mechanisms and thought processes of four different species of lemur.
Recommended as the most conflict-prone group at the center, the familial pairing of Halley soon became the target of my interest. During the time of my observations this summer, this family was in the middle of a power dispute. Varecia rubra is a matriarchal species and as such mother Halley stood as the dominant source of power. However, I had been informed upon arrival that her daughter Helene was challenging her for dominance- a conflict that was causing a great deal of physical altercations. The family group also included Helene’s twin sister Harriot, and father Ravo, who both possessed submissive personalities more akin to followers rather than leaders. Throughout my sessions of watching the group, I was fascinated to see the many situations that provoked Helene and Halley to engage in physical altercations and fight-off for dominance. However, one particular strategy of Helene’s became of immense interest to me. After most physical altercations with her mother, she would go to her father and sister and engage in activities of support and affection. In most cases, this was exhibited as communal grooming or cuddling. Helene employed this strategy many times, and it seemed to be her way of seeking justification for her actions from her father and sister. By appealing to the remainder of the family group as a caring social figure, Helene could further alienate her mother from a position of power and get her sister and father to take her side in any physical altercation.
This power dynamic and people-pleasing strategy reminded me of a people’s revolution in American politics where a revolution begins by rallying a majority of the populace around a leader. Because this new leader has the defense-based support of a majority within the community, they are able to overthrow the current leader and a new regime is established. Being heavily interested in becoming a game theorist, I began to wonder if there was some way to create a quantitative model showing the logic of this strategy. If I formulated a game about this concept of revolution and either obtaining or maintaining a regime, I might be able to learn more about how to model changes in power and how political strategies have evolved over time.
As such, this paper will seek to create a quantitative model that exemplifies the powerful strategy of obtaining societal members to back a regime. This model system will then be utilized alongside a game of Revolution in which players are challenged to create and maintain the dominant regime for the longest period of time possible. Together, this quantitative game- theoretic model should mimic the pattern of revolutionary strategy as seen both in the natural world to draw parallels between non-human primate defense strategies and those of the current political world.
A Quantitative Model
Introduction
The most difficult part of creating a quantitative model for a political situation is the complexity of animalistic behavior. Whether those animals be a family pairing of Varecia rubra or Homo sapiens, the primary challenge is that of making the complex simple. A quantitative model does not seek to necessarily depict every small decision or behavior; instead, it seeks to strip a strategic situation down to its infrastructure and outline the core decision making process in the event. This is how the following quantitative model will aim to address this latter goal. The model seeks to outline a game-like structure to reflect the dominant strategies of a social revolution. To achieve this, the model identifies a new conceptualization of a win, implements a point-based system and a following score to measure the number of individuals supporting a given leader at any particular time, and an overall strength score to indicate how strong a particular regime is. These four components, combined with the discrete rules of the game, create the proposed quantitative model.
The Binary State-Dependent Win
One of the most difficult parts of creating a game that models political interactions is that there is often no explicit win that terminates the game, instead political choices and decisions about fighting for power will continue on. As such, in a revolution, winning is a state- not a final decision that ends the game. As with any revolution, the main objective is to obtain power over the group of other players. This means that the person in power at any given time is winning while all other players out of power are in a state of losing (at the beginning of the game, however, no one will be winning as a regime has yet to be established). However, this state of winning can be stolen by another player who achieves the desired power over the other players. This illustrates the difficulty, specifically winning is a state that changes hands as time plays out in the game. This makes “winning” dependent on a certain window of time. This is where a new conceptualization of a winning comes into play- one that depends on the state at hand: The State-Dependent Win.
Next comes the step of modeling a state-based concept of winning. In this model, the State-Dependent Win is crafted to be binary in nature. This is because at any given time an individual is either winning (a value of 1) or loosing (a value of 0). Binary remains the traditional way of mathematically representing wins versus losses. Through use of a piecewise function f(x), this binary win/loss structure can be graphed over time for any n number of players.
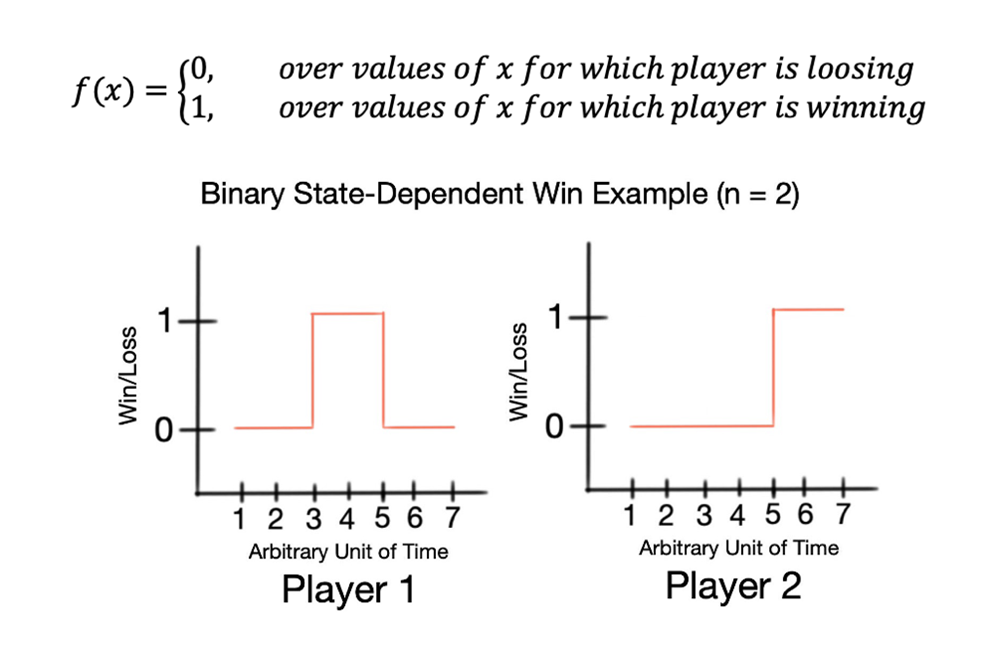
Figure 1: The two graphs of a Binary State-Dependent Win for players 1 and two in a two- person game of Revolution. The graph also distinguishes how no player is imbued with a regime at the beginning of the game- each player must fight for the establishment of their own regime.
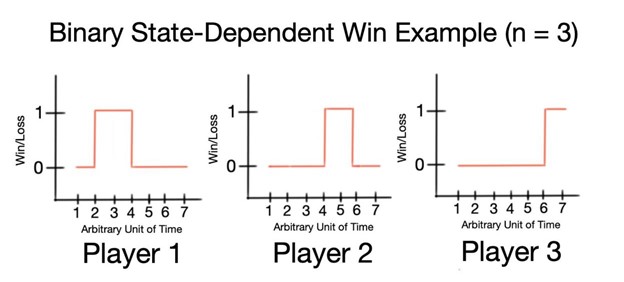
Figure 2: The three graphs of a Binary State-Dependent Win for players 1, 2 and 3 in a three- person game of Revolution. The graph also distinguishes how no player is imbued with a regime at the beginning of the game- each player must fight for the establishment of their own regime.
As shown above in figures 1 and 2, the Binary State-Dependent Win values can be graphed across any arbitrary unit of time. Since there can only be one individual winning at any given time (the regime in power holds only one dominant leader as per the rules, described later), the graphs depict only one player as existing in the “winning” state with a value of 1 at any time. By understanding a win as a state and not the final end-objective of a game, the capacity for modeling real life scenarios expands. The Binary State-Dependent win tells us more than anything else not who wins a game, but who is winning at any point in time. Yet, in any given game with a discrete amount of time, there has to be an inevitable winner. So how do we conceptualize an overall win amidst a discrete amount of time from a game with a state of winning?
Time-Sensitive State Dependent Win Coefficient
In most games, a win is determined when a game comes to an end. However, in Revolution, this is not the case, and thus, it can continue to occur for any period of desired time. That said, when this period of time comes to an end, it is essential to turn the state- dependent conceptualization of “winning” into a solidified win. There remain two distinct possibilities for determining a final win. First off, whoever exists in the state of winning amidst a discrete amount of time is considered the consistent winner. This will be referred to as the State Final Win. This however is a difficult conceptualization of a win because although an individual could be in the state of winning when time has elapsed, they may not have been in a position of winning for most of the discrete time interval in question. Being able to obtain a position of power and then maintain it for a substantial duration of the game signifies strength and capability. This is because the dominant strategy for someone in the winning state is to maintain their power. As such, a win could also be conceptualized as the person who was in a winning state for the
greatest amount of time. As this reflects a stronger defense strategy, this is the form of win that will be adopted by this model.
In order to determine who is the winner of a particular game, one must be able to create a coefficient that measures who has been in the state of winning for the largest amount of time. This can be done by taking the sum of all win/loss 1 or 0 values for all units of time and then dividing those values by the final finite amount of time to create a Time-Sensitive State Dependent Win Coefficient.

Figure 3: The follow equation depicts the calculation of the Time-Sensitive State Dependent Win Coefficient: the division of the sum of all win/loss values (the f(x) values of each Binary State- Dependent Win graph) by the sum of the final amount of elapsed time xn.
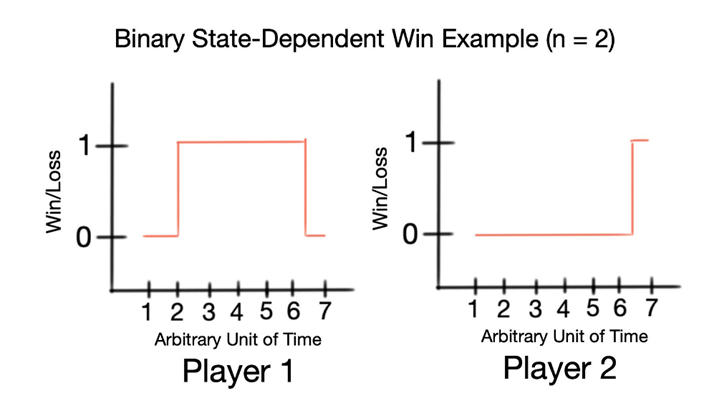
Figure 4: In the graphs depicted above, Player 2 would win under the State Final Win model despite Player 1 having maintained a successful regime for a longer period of time. By calculating the Time-Sensitive State Dependent Win Coefficient, Player 1 wins, accurately rewarding the player with the stronger strategy and higher revolutionary capabilities.
The Time-Sensitive State Dependent Win Coefficient simply requires that the game has a finite amount of time, whatever that time is determined to be. This finite quantity of time is normally determined at the termination of the game. However, the coefficient’s calculation could also be done in real time, receiving data about the current sum or win/loss values and the ongoing quantity of time. Thus, it is possible to know who would win the game at any particular moment should the game hypothetically stop at a given point in time, another benefit of the Time- Sensitive State Dependent Win Coefficient.
The formation of the win in this manner allows one to determine the winner of the game on the basis of the individual strong enough to maintain their regime for the longest amount of time, more accurately rewarding the player whose regime was stronger. This provides a solid quantitative system, but it is essential to dive deeper into understanding the strategies of the Revolution game even more, which the study will implement with the implementation of an Innate Point-Based Value System and Following Score.
Innate Point-Based Value System and Following Score
When witnessing a revolution of any kind whether within a Varecia rubra familial group or in history, the dominant strategy that appears to emerge is what I will refer to as the “strength in numbers” strategy. This is the concept that winning a revolution or maintaining a regime requires obtaining the largest number of supporters. The game of revolution exemplifies a few asymmetric strategies. After the beginning of the game (when all players are struggling to take power and the first regime is eventually established) there is one individual winning and one individual loosing throughout the remainder of the game. As such, the strategies of the player winning will differ from those of the remaining players who are losing. The winning player seeks to maintain their regime while the loosing players look to obtain a regime. Although the perspectives of the winning and loosing players are asymmetric, they are dependent on the number of followers each player had in their regime in my observations of Varecia rubra. Therefore, the player with the largest number of followers would be the one to either maintain their regime or establish a new one. My goal in creating a quantitative model it to create a point-based value system that accentuates this “strength in numbers” strategy.
As seen with the lemurs, each individual animal has about the same defense capabilities. Unlike other primates, the male or female Varecia rubra are not drastically large or smaller in comparison to their opposite sex. While female Varecia rubra are normally the only members of a community to engage in aggressive behavior, the aggressive capabilities of each individual lemur are quite evenly matched. As such, on a simplified level, each lemur contributes about the same tactical capabilities as all others. From a game theoretic standpoint, this means that each member of n participants will have an equal potential defense- based contribution to any regime they lead and/or support. Therefore, in the quantitative model, each player will possess an innate value of 1, creating the game’s Innate Point-Based Value System. This value of 1 represents the defense capabilities of each player. Because all players have a simplified equal capacity to defend, each player has a value of 1. Innate Point-Based Value System will let one better understand and model the dominant “strength in numbers” strategy in the game of Revolution.
As described with relation to the “strength in numbers” strategy, observations show that the player with the highest number of followers is the one most likely to either obtain the spot as the dominant leader or be the leader who maintains the regime already in place. As such, it is important to have a value that represents the number of followers any given player ascending to a leadership position obtains. This is important on an analytical level because it allows one to assess whether or not there is a statistically significant correlation between whoever is “winning” and whoever has the highest number of followers. When a player actively decides to support a given regime and become another player’s follower, they give their Innate Point-Based Value to said leader. This is because pledging allegiance to a cause, leader, and/or regime often means
being available to defend the group of individuals you are allying yourself with. Because the Innate Point-Based Value represents the defense capabilities of an individual, it is consistent with the behavior of allying yourself with a regime to give your value of one and therefore your defense capabilities over to your ally. As such, one can create the Following Score, which is the number of Innate Point-Based Values given to a regime leader, indicating the level of defense- based support they have.
The Innate Point-Based Value system and the Following Score, one can accurately model how defense-based support is given to a regime and how said support determines a player’s state of “winning” or “loosing”. These two systems are essential to understanding the strategies of each player and regime on a quantitative level as the game progresses.
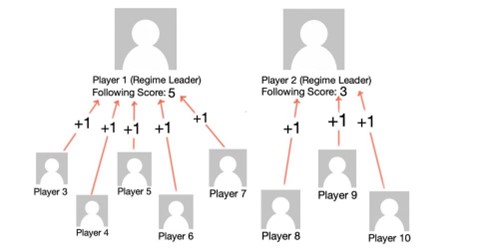
Figure 5: The above diagram depicts how the Innate Point-Based Value System and the Following Score are utilized together to represent how alliances strategically play out across the game as a way of analyzing how followings contribute to being in a “winning” or “loosing” state. This is drawn with an n=10 person game.
The Strength Score
Throughout this model, there has been a prominent discussion as to strong versus weak regimes. Earlier, it was discussed how the Time-Sensitive State Dependent Win Coefficient was a more beneficial win model than the State Final Win due to its ability to represent a stronger regime more so than a regime that just entered into a state of winning. The Time-Sensitive State Dependent Win Coefficient does this by quantifying for how much time a person upheld their regime. Similarly, the Following Score for an individual regime leader show not only how many individuals are allied with their regime but also how much defense power they possess. As such, strength can be considered as a measure of the sturdiness of a regime combined with the defense-based power the regime holds. With this in mind, the model also proposes a coefficient of strength: The Strength Score. This Strength Score will be the value of the Time-Sensitive State Dependent Win Coefficient multiplied by the Following Score of an individual, quantifying the strength provided.
Equation: 𝑆𝑡𝑟𝑒𝑛𝑔𝑡ℎ 𝑆𝑐𝑜𝑟𝑒=𝑇𝑖𝑚𝑒−𝑆𝑒𝑛𝑠𝑖𝑡𝑖𝑣𝑒 𝑆𝑡𝑎𝑡𝑒 𝐷𝑒𝑝𝑒𝑛𝑑𝑒𝑛𝑡 𝑊𝑖𝑛 𝐶𝑜𝑒𝑓𝑓𝑖𝑒𝑛𝑡 ∙ 𝐹𝑜𝑙𝑙𝑜𝑤𝑖𝑛𝑔 𝑆𝑐𝑜𝑟𝑒
Example:
0.56 = Time-Sensitive State Dependent Win Coefficient
11 = Following Score
Strength Score = 0.56 ∙ 11 = 6.16
Figure 6: The equation and example calculation for the Strength Score.
Thus, whoever possesses the highest strength score can be considered to have the strongest regime.
Rules of the Revolution Game
With a win defined and a point system in place, it is now important to outline the general rules for the Revolution game:
(1) The main objective of the game is to establish and maintain the dominant regime over a group of individuals. Any player can be the leader of a regime. The next best alternative to be the leader of a regime is being a member of the dominant and longest lasting regime.
(2) Each individual is given an innate point value of 1. If this game is being played in person, this value can take the form of a physical object such as a single dollar bill or a marble.
(3) Open communication between all participants is the allowed form of defense. However, no aspect of physical fighting or murder exists in the game. Participants must only use their words to defend themselves or other players in the game.
(4) As players begin to rally other players around leaders to create a regime, all individuals who follow these regimes give their innate point value over to the leader of the regime.
While these rules are inherently simple and sparse, the interest lies in watching the many verbal and behavioral strategies that develop to coerce new members into joining a particular regime. The rules are designed to be open-ended as to mimic the immense expanse of behavioral choices allowed in real-life, making the game as realistic as possible. When providing an overview of the model, it is important to go back to the model’s original purpose: to create a quantitative model that helps asses the social and behavioral strategies of a revolutionary situation with particular focus on alliances, the strength and the lifetime of a regime. As the power dynamics change and regimes rise and fall, one can assess who is winning through use of the Binary State-Dependent Win and who wins through the Time-Sensitive State Dependent Win
Coefficient. The effectiveness of particular strategies can be assessed through use of the Following Score (which exists due to the implementation of an Innate Point-Based Value System) and the Strength Score, showing how all elements of the quantitative model tie together to achieve the desired main purpose.
A Look Forward: Modeling Political Revolutions and Human Politics
As touched upon in the Behavioral Inspiration section of this paper, this “strength in numbers” people’s revolution strategy is one prominently observed throughout human history. From Revolutions in America and France to China, the tactic of winning over the majority of support in a populace is a strategy seen consistently throughout history. As identified in this quantitative model, there is a discrete strategic advantage to winning over the support of a populace. Many revolutions (such as the American and French Revolutions) involve large parties of the public overthrowing a monarchical or tyrannical government an implementing a democracy. Because democratic ideals are based on electing leaders from immense public support, this strategic advantage of the “strength in numbers” strategy may provide some basis for the implementation of democracies. Therefore, it is interesting to see a model indicate the strategic strength of democracy. As such, I believe my model might have the potential to analyze current revolutions occurring around the world. While the work is based on an evolutionary dynamic, it would be interesting to see if this strategy of “strength in numbers” emerges when being played by a group of humans. Similarly, I would be fascinated to see if measures such as the Time-Sensitive State Dependent Win Coefficient, Following Score, and Strength Score could be used to predict which parties were going to win a current day revolution at any point in time.
Going forward, I’d like to study the application of this model on current day revolutions as well as having humans enact the Revolution game in a controlled situation that is under observation. I hope to learn more about the validity of this “strength in numbers” strategy in modern day politics, as I feel better understanding its extreme advantage could help decipher the motives behind many defense-based policies in our world today.
Sources
Aili, Han. December 7, 2012. A Strategy Based on Probability Theory for Poker Game. The Institution of Engineering & Technology.
Belletto, Steven. June 2009. The Game Theory Narrative and the Myth of the National Security State. Johns Hopkins University Press.
Berkman, Robert M. January 2004. The Chess and Mathematics Connection: More Than Just a Game. Mathematics Teaching in the Middle School
Boros, Endre. February 28 2012. On Nash equilibria and Improvement Cycles in Pure Positional Strategies for Chess-Like and Backgammon-Like n-Person Games. Elsevier: Discrete Mathematics.
Cooke, Charlie H. January 2019. Probability Models for Blackjack Poker. Elsevier: Computers & Mathematics with Applications
Euwe, M. 2016. Mathematics- Set-Theoretic Consideration on the Game of Chess. World Scientific: New Mathematics and Natural Computation.
Gilpin, Andrew. October 2007. Lossless Abstraction of Imperfect Information Games Journal of the ACM.
González-Díaz, Julio. 2010. An Introductory Course on Mathematical Game Theory. American Mathematical Society.
Gurvich, Vladimir. 2013. On Nash-Solvability in Pure Stationary Strategies of the Deterministic n-Person Games with Perfect Information and Mean or Total Effective Cost. Discrete Applied Mathematics.
Hope, Linda. March 1994. Mission Possible: Computers in Chess and A-Level Mathematics. The Mathematical Gazette.
Hoda, Samid. May 2019. Smoothing Techniques for Computing Nash equilibria of Sequential Games. Mathematics of Operations Research.
Javarone, Marco Alberto. November 17, 2016. Modeling Poker Challenges by Evolutionary Game Theory. MDPI.
Makridakis, Spyros. March 27, 2018. Statistical and Machine Learning Forecasting Methods: Concerns and Ways Forward. Public Library of Science.
Powley, Edward J. Information Capture and Reuse Strategies in Monte Carlo Tree Search, with Applications to Games of Hidden Information. Elsevier: Artificial Intelligence.
Schelling, Thomas C. 1966. Arms and Influence. New Haven and London Yale University Press.
Slezak, Diego Fernandez. March 2, 2018. An Entropic Barries Diffusion theory of Decision- Making in Multiple Alternative Tasks. Public Library of Science: Computational Biology.
Velupillai, Vela K. April 2 2018. Proofs and Predictions in Human Problem Solving. Springer Science + Business Media LLC.